成果推介 | 我院教师倪禾在Expert Systems and Applications发表论文
发布日期:2023-04-12 供稿人:夏连峰 浏览次数:759
近日,我院教师倪禾在 Expert Systems with Applications 发表题为Contextual combinatorial bandit on portfolio management 的论文。
Expert Systems with Applications 专注于交流世界各地工业、政府和大学应用的专家和智能系统的相关信息。该期刊发表的论文涉及专家和智能系统技术,以及各个领域的应用。根据爱思唯尔的数据,该期刊目前是人工智能领域的一流期刊,SCI 1区期刊 。
Portfolio optimization is a classic problem in finance, and assumptions are generally accepted about the stochastic dynamics of prices and market information. The Linear Upper Confidence Bound (LinUCB) algorithm based on bandit learning is a typical reinforcement learning approach that can be a data-driven approach to the portfolio problem. Most investors may have inconsistent preferences for different assets; therefore, the reward function needs to be designed to balance exploration (allocating part of the investment to searching among other alternative portfolios) and exploitation (concentrating the investment on the historically best portfolio). The study consists of developing a two-stage investing strategy that uses the supervised adaptive decision tree approach to build a pool of candidate portfolios and the reinforcement LinUCB algorithm to derive a portfolio update rule with respect to a specific utility function. The two-stage strategy has been tested on CSI300 constitute stocks listed on the Shanghai Stock Exchange. The accumulated returns, as well as other performance measures (e.g., maximum drawdown, Sharpe ratio, and information ratio) at different testing periods achieved by the model, can well outperform the benchmark index.
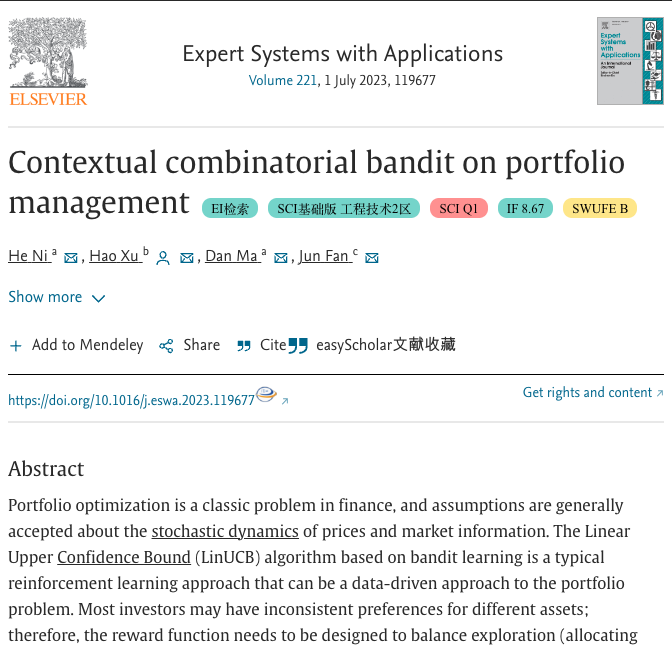
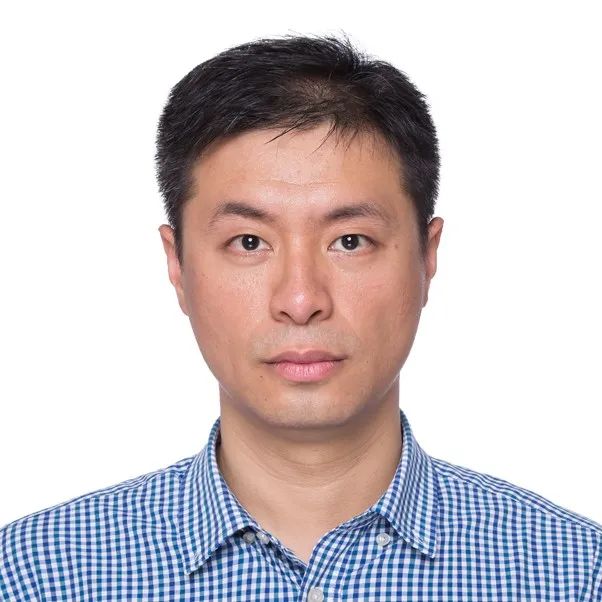
倪禾 浙江杭州人,分别在英国曼彻斯特大学、英国谢菲尔德大学、西安电子科技大学获得博士、硕士和学士学位。浙江工商大学金融学教授,现任泰隆金融学院副院长。主要研究领域有:金融时间序列分析、量化交易、神经网络技术在资本市场的应用。

